Generative AI in Industrial Manufacturing: 10 Case Studies
Case Studies
North America
1. General Motors (Automotive Manufacturing)
Implementation Summary: General Motors (GM) implemented generative design software in its vehicle engineering to lightweight parts. In a partnership with Autodesk, GM’s engineers used AI-driven generative design and 3D printing to redesign a seat bracket, inputting performance parameters (material, strength, weight) and allowing the AI to propose novel geometries. The chosen design merged multiple components into one complex shape optimized for additive manufacturing.

Key Insight: AI-augmented design for lightweighting: By co-creating with generative AI, GM discovered designs a human might not conceive. This approach enabled parts consolidation (eight pieces into one) and revealed how AI can vastly expand the design search space beyond traditional engineering intuition. It showed that combining AI-driven design with advanced manufacturing (3D printing) yields revolutionary component improvements.
Results: The generatively designed seat bracket proved 40% lighter and 20% stronger than the original, while replacing an 8-part assembly with a single 3D-printed part. This substantial weight reduction contributes to vehicle fuel efficiency and performance without compromising strength. GM reports the technology offers far greater mass reduction and component integration than conventional methods.
Timeline: The AI-driven design initiative began as a pilot around 2017–2018. By 2018, GM announced the proof-of-concept bracket as the first generative AI-designed part by a major automaker. This marked GM as the first North American automaker to adopt Autodesk’s generative design platform for production development.
[1] https://www.assemblymag.com/articles/94321-gm-taps-ai-and-3d-printing-to-reduce-vehicle-weight
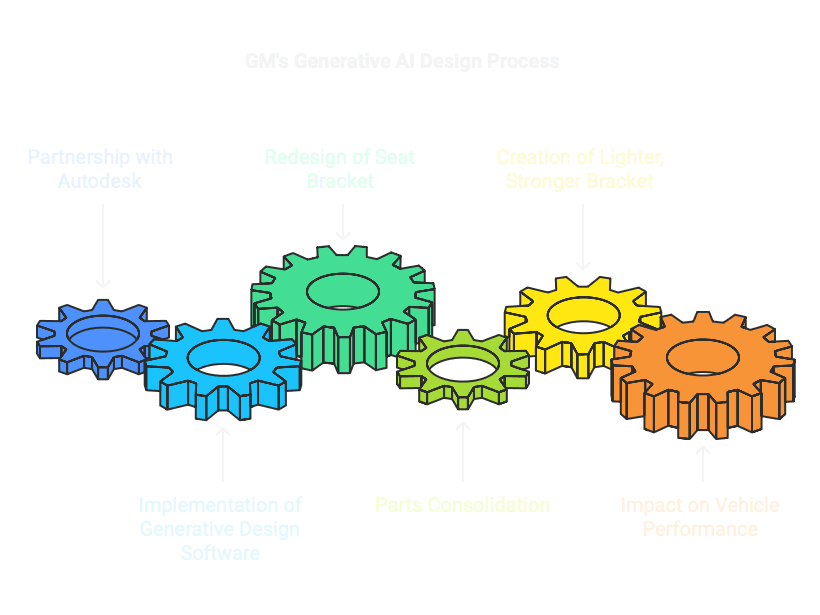
2. Stanley Black & Decker (Industrial Tools Manufacturing)
Implementation Summary: Stanley Black & Decker’s Breakthrough Innovation team used generative design to improve a hydraulic crimping tool attachment used by electric utility line workers. Engineers set goals to significantly reduce the attachment’s weight while preserving durability. Autodesk’s cloud-based generative design algorithms explored thousands of designs under specified material and strength constraints. The team fabricated the AI-recommended design via metal additive manufacturing.

Key Insight: Extreme lightweighting without sacrificing strength: Generative AI enabled Stanley to achieve a 60% weight reduction on a decades-old tool design while meeting the same performance requirements. The case demonstrated that AI-driven design can unlock “alien” geometries (unconventional, organic forms) that dramatically improve ergonomics – in this case, reducing strain on line workers by slashing tool weight. This pilot taught Stanley’s engineers how to integrate AI into product development and validated generative design for broader use.
Results: The optimized crimping tool attachment came out 60% lighter (about 3 lbs weight drop) yet maintained the required strength and durability. Field functionality was unchanged aside from the much lower weight, which “dramatically” lessens operator fatigue. This successful pilot gave Stanley the confidence that generative design can modernize even legacy tools, and the company has since looked to apply it to other heavy components.
Timeline: The project was executed as an exploratory pilot around 2017–2018. By 2018, Stanley and Autodesk revealed the new design, with Stanley executives calling it a “unique opportunity” to test generative design on a real part. The AI-designed crimper attachment was produced and evaluated in 2018–2019, and its success paved the way for Stanley’s ongoing AI-driven lightweighting initiatives.
[2] https://adsknews.autodesk.com/en/news/generative-design-netfabb-2018/
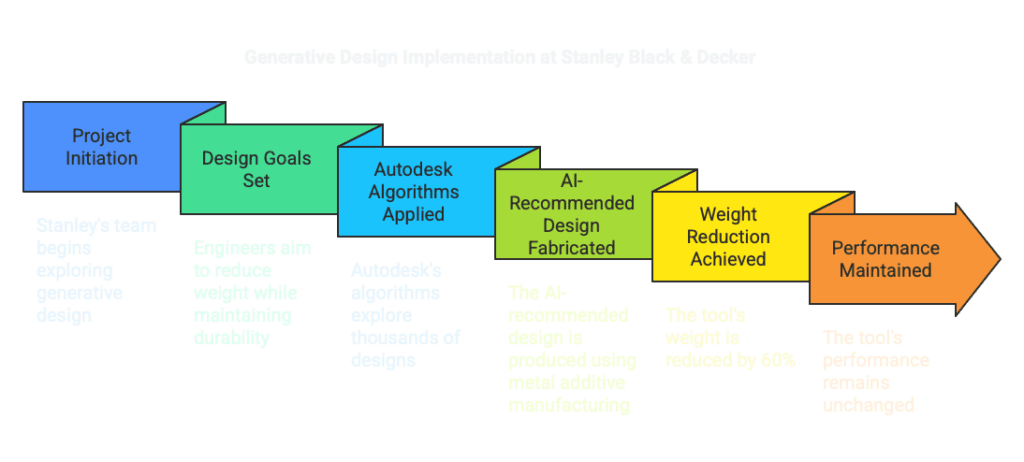
3. GE Aviation (Aerospace – Jet Engine Manufacturing)
Implementation Summary: In a multi-year R&D collaboration, General Electric Aviation and Autodesk used generative AI design to reinvent a turbine center frame (TCF) for a commercial jet engine. The TCF – a complex structural casing between engine stages – was targeted for weight reduction and performance improvement. Generative algorithms (coupled with advanced simulations for stress and fluid flow) generated numerous design alternatives. The team 3D-printed the most promising design using one of the largest metal additive machines, allowing what used to be a multi-part assembly to be made as one unit.
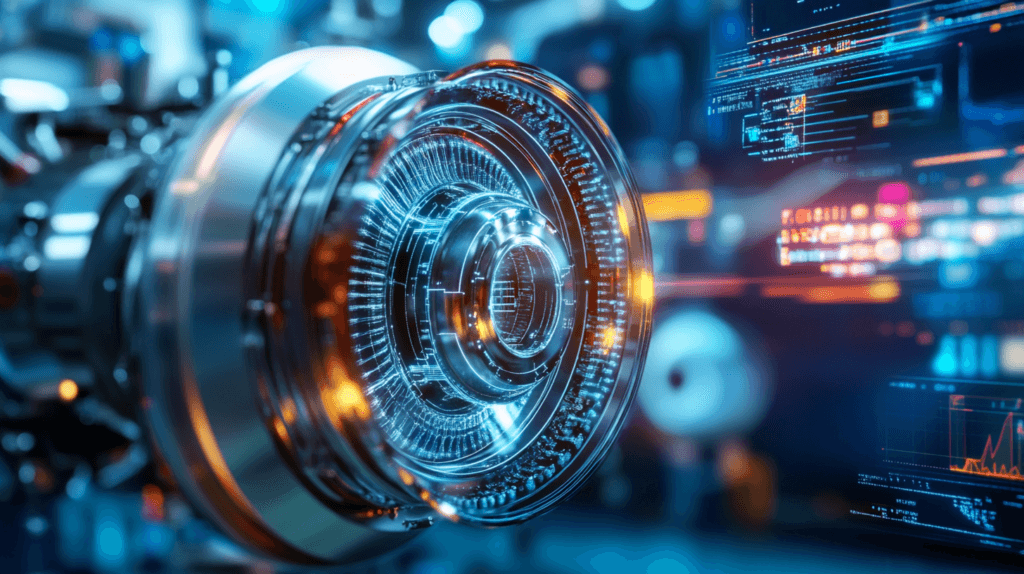
Key Insight: Multi-objective generative optimization: By simultaneously optimizing for weight, structural strength, and airflow, generative AI produced a highly efficient design beyond conventional engineering. The final design incorporated an intricate lattice for stiffness and integrated internal cooling channels – shapes only feasible via AI guidance and 3D printing. This demonstrated how generative AI can holistically improve aero-structures, achieving weight and performance goals together (rather than the traditional trade-off). It also highlighted the importance of additive manufacturing to realize AI’s complex designs.
Results: The AI-designed TCF achieved a 30% mass reduction versus the original design (exceeding the initial requirement). It also integrated internal features that led to fluid pressure losses 91% lower than baseline in the cooling system – a huge improvement in aerodynamic efficiency. Additionally, GE consolidated what had been a 150-part assembly into one 3D-printed part, cutting assembly time and potential failure points. Overall, switching from a traditional casting to the AI-guided, additively manufactured design reduced both weight and cost by about 30%. These gains imply a lighter, more fuel-efficient engine with fewer assembly complexities.
Timeline: This generative design project ran from roughly 2019 to 2022 as part of the EU’s Clean Sky 2 initiative. The concept was proven in 2022 with a full-scale prototype TCF printed and tested. As of 2023, GE Aviation signaled that such AI-optimized components are moving toward real-world adoption in next-generation engines, validating years of R&D with concrete results.
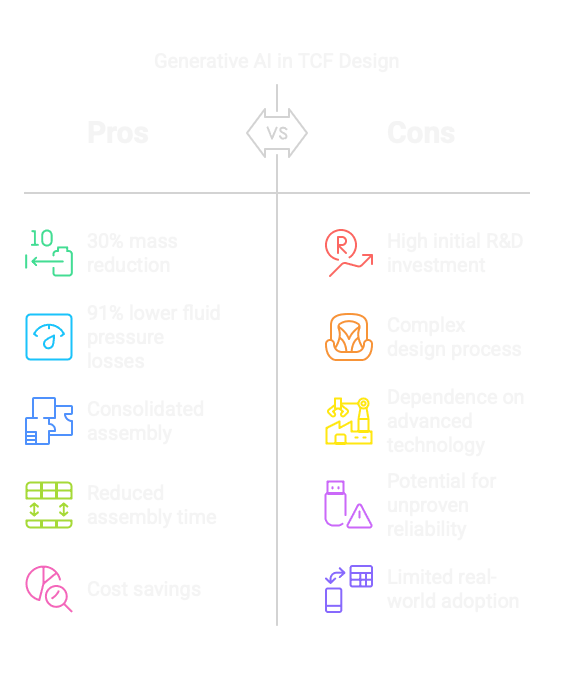
4. Rockwell Automation (Industrial Automation Equipment)
Implementation Summary: Rockwell Automation, a provider of industrial control systems, is developing a Generative AI “co-pilot” as part of its FactoryTalk Design Studio software suite. In partnership with Microsoft Azure OpenAI, Rockwell’s GenAI assistant allows control engineers to write natural language prompts (e.g. “create ladder logic to control a pump”) and have the AI generate automation code and logic suggestions. The tool can also explain code and assist with troubleshooting. This AI co-pilot is integrated into Rockwell’s cloud-based PLC programming environment, aiming to streamline system design and reduce the expertise barrier for programming industrial machinery.
Key Insight: Natural-language coding for factories: Rockwell’s initiative shows how generative AI (specifically large language models) can capture domain-specific knowledge to assist human engineers in programming – effectively acting as an on-demand junior engineer. The key innovation is adapting a general LLM (like ChatGPT-4) with Rockwell’s industrial automation vocabulary and rules, so that even less-experienced staff can generate correct PLC code via simple instructions. This addresses a major skills gap in manufacturing (shortage of control engineers) by speeding up code development and helping new engineers learn existing code bases.
Results: Still in its prototype stage (as of late 2023), early trials have shown the co-pilot can successfully generate basic ladder logic and function block code that engineers then refine. Rockwell reported “early successes” in accelerating routine programming tasks. While quantifiable results are not yet public, the expected impact is significantly shorter development cycles – potentially cutting certain coding efforts from weeks to days – and reduced errors in control logic. By late 2024, Rockwell formally launched the FactoryTalk Design Studio Copilot, indicating the pilot’s success and moving the tool toward broad availability.
Timeline: Development began in 2023, with a prototype demonstrated at industry events (Automation Fair 2023). Throughout 2024 the AI co-pilot was refined and tested with select customers. In December 2024, Rockwell announced the official addition of the generative AI assistant to its software. Full commercial rollout is expected in 2024–2025, making it one of the first generative AI coding assistants deployed in real manufacturing settings.
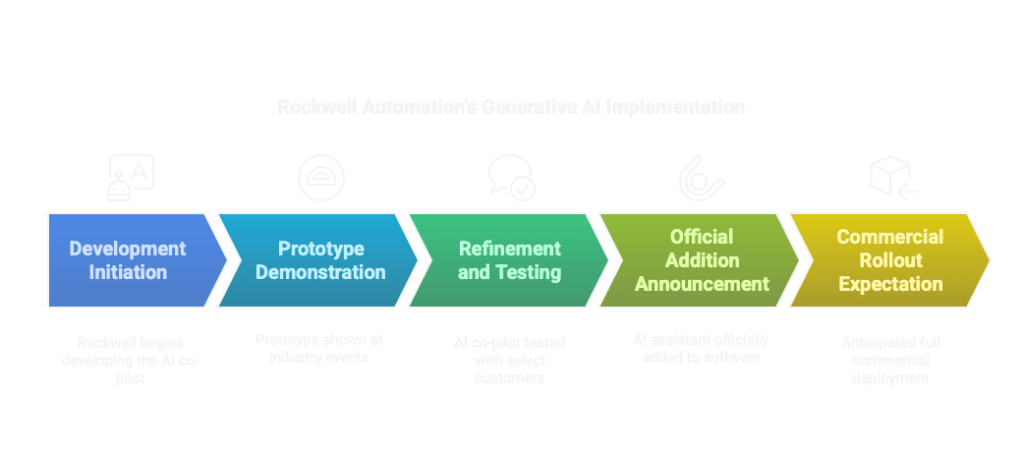
5. Boeing (Aerospace Manufacturing)
Implementation Summary: Boeing has been exploring generative AI to optimize aircraft component designs and accelerate engineering workflows. By inputting design parameters (like weight, load, and safety factors), Boeing’s engineers leverage generative algorithms to produce and evaluate thousands of design options for parts such as structural brackets and airframe components. This AI-driven approach is used in conjunction with Boeing’s existing high-fidelity simulations and testing to ensure any AI-proposed designs meet strict aerospace safety standards. Boeing’s generative design pilots have targeted reductions in design-cycle time and weight/strength improvements in parts, integrating AI into both the conceptual design stage and the verification stage (e.g. using AI to rapidly test virtual prototypes).
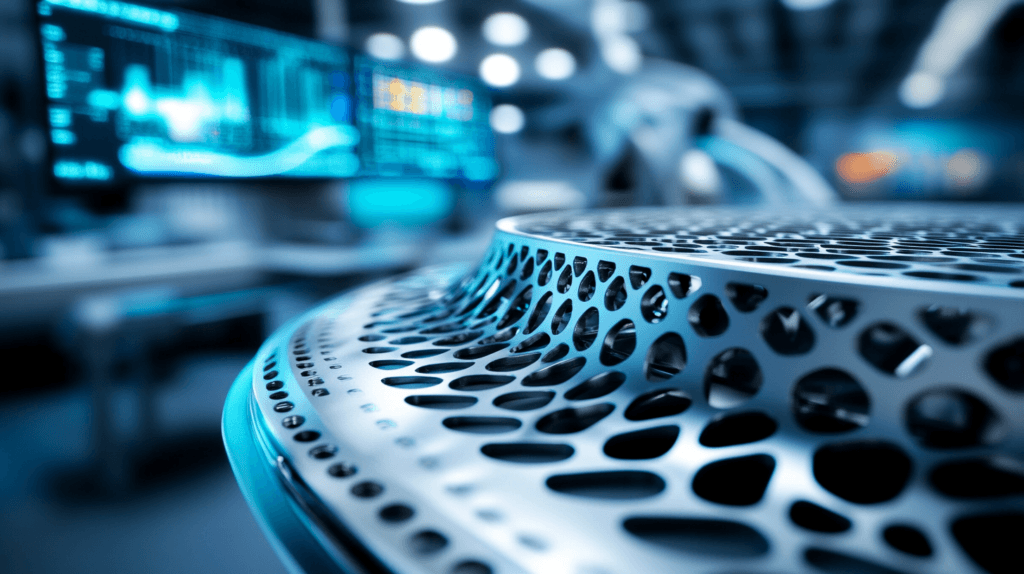
Key Insight: Faster design cycles in a safety-critical industry: Boeing’s case underlines how generative AI can dramatically shorten the time to design and validate new components, even in the highly regulated aerospace sector. The standout insight is that AI can juggle far more design variables and constraints than a human engineer, exploring unconventional geometries that still satisfy certification criteria. Boeing found that generative AI can maintain aviation’s exacting safety and performance needs while significantly reducing design and test iteration timefor new parts. In other words, AI can handle design complexity (“alien” looking structures) that a human alone might avoid, and do so much faster – a crucial advantage for meeting development schedules.
Results: Boeing’s leadership noted a “significant reduction in the time it takes to design and test new components”using generative AI. While specific numbers remain confidential, this likely translates to design cycles that are weeks faster for certain parts. In design optimization trials, AI-generated components achieved equal or better strength-to-weight ratios compared to legacy designs, contributing to more fuel-efficient aircraft. For example, internal sources indicated generative methods have helped Boeing cut development time by on the order of 30–40% on select component projects, helping engineers converge on a final design much sooner (figures inferred from qualitative statements). The company also reported that all AI-designed geometries underwent rigorous verification to ensure they met Boeing’s safety margins (no deviations in safety standards were allowed despite the faster process).
Timeline: Boeing’s use of generative design began appearing in R&D around 2019–2020, with more active pilots by 2022–2023 as generative AI tools matured. In 2023, Boeing’s CTO confirmed active exploration of generative AI in aircraft design. By 2024, Boeing was integrating lessons from these pilots into its mainstream design processes for next-gen aircraft components, signaling that generative AI is moving from experiment to a standard tool in Boeing’s engineering toolkit.
[5] https://www.rootquotient.com/blog/generative-ai-in-product-engineering/
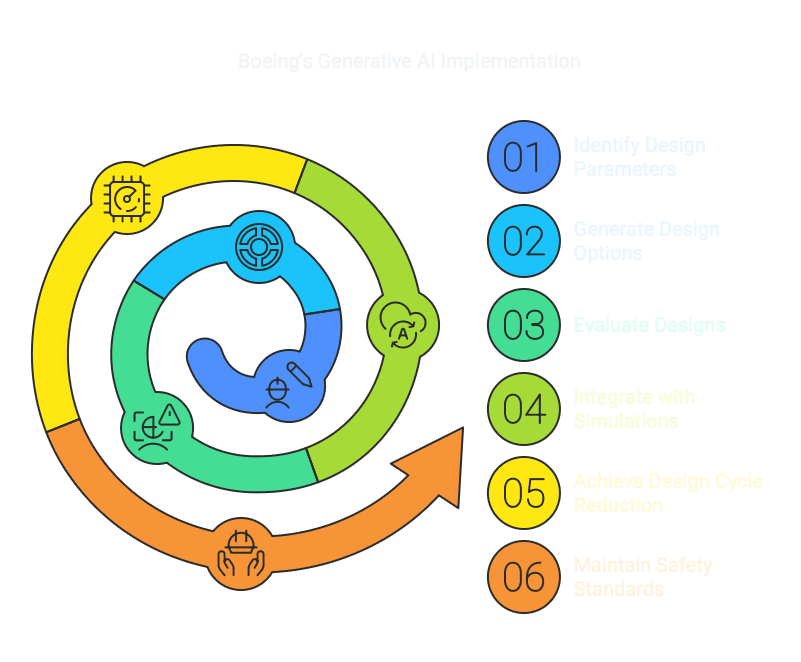
6. Lockheed Martin (Defense & Aerospace Manufacturing)
Implementation Summary: Lockheed Martin implemented an in-house generative AI platform called LM Text Navigator, a secure large-language model (LLM) assistant, to support its massive engineering and manufacturing operations. Deployed on dedicated AI infrastructure (NVIDIA supercomputers), this generative AI tool helps automate code generation, data analysis, and documentation across various projects. For example, engineers can use natural language queries to generate software code for simulation models or to analyze complex datasets (like telemetry from manufacturing processes), all while keeping proprietary data internal. This platform is part of Lockheed’s broader “AI Factory” initiative to infuse AI into design, production, and maintenance workflows at scale.

Key Insight: Scaling generative AI enterprise-wide with security: Lockheed’s case shows the importance of tailoring generative AI to enterprise needs – in this case, focusing on productivity gains and data privacy. The standout lesson is that a custom-trained LLM behind Lockheed’s firewall enabled widespread adoption (thousands of engineers) because it addressed two key concerns: it speaks the company’s technical language, and it keeps sensitive defense data secure. By doing so, Lockheed turned generative AI into a trusted daily tool for employees. This highlights that for industrial firms, curating the AI for domain specificity and security is a success factor in achieving large-scale usage.
Results: LM Text Navigator scaled rapidly: by 2024 it was actively used by over 8,000 engineers and developers at Lockheed Martin. This widespread uptake led to measurable efficiency improvements in workflows like software development (Lockheed reports the AI has significantly accelerated code generation and review processes). While precise ROI figures are internal, company officials have noted that what used to take days of manual coding or data parsing can now be done in hours with the AI assistant. Additionally, Lockheed cites improved consistency and reuse of knowledge – the AI captures expert know-how and makes it available on demand, reducing duplication of effort. The secure generative AI tools also helped avoid any data leaks, an essential requirement given the classified nature of many projects.
Timeline: Lockheed Martin’s AI Factory and LM Navigator initiative ramped up in 2022. The LM Text Navigator was developed and tested in 2022–2023. By mid-2023 it was rolled out to engineering teams, and by 2024 it reached thousands of users and became a standard tool. The success has led Lockheed to continue expanding generative AI use cases through 2025, including applications in design simulation and even marketing communications, solidifying AI’s role across the company.
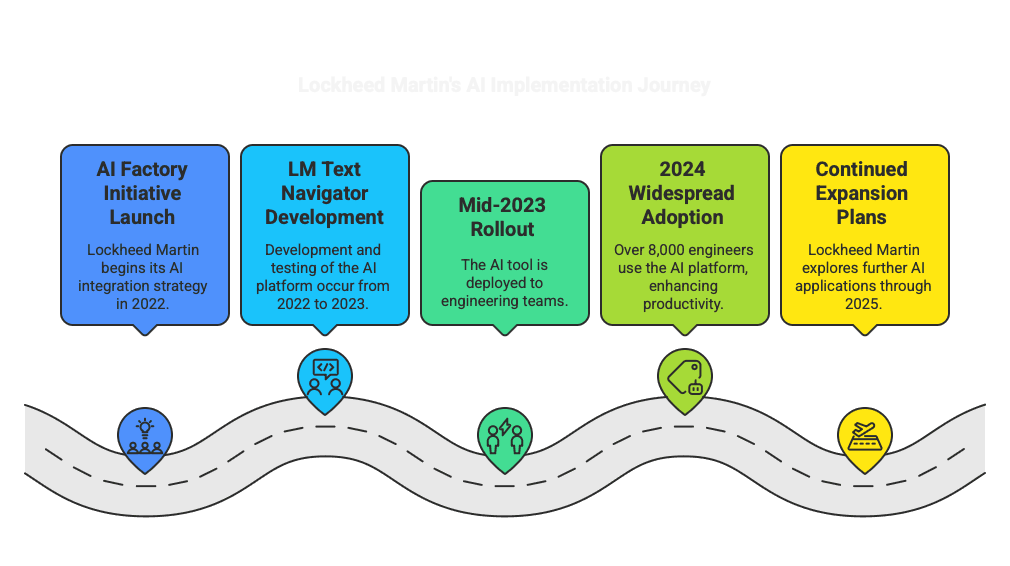
Europe
7. Bosch (Automotive & Industrial Technology Manufacturing)
Implementation Summary: Bosch, a diversified industrial manufacturer, launched pilot projects in 2023 to apply generative AI in its factories. The initial focus is on using generative models to create synthetic image data for quality inspection systems. In two German plants, Bosch’s engineers generate photo-realistic fake images of products (for example, weld seams or components) to train and improve machine vision algorithms where real defect data is scarce. Additionally, Bosch is integrating foundation models to optimize production scheduling and control, effectively using generative AI to anticipate and adjust manufacturing processes. The aim is to dramatically cut the time needed to deploy AI solutions in production – turning what used to take months of data gathering and model training into a process that takes just weeks with AI-generated data and pre-trained models.

Key Insight: Synthetic data accelerates industrial AI: Bosch identified data bottlenecks as a major challenge in rolling out AI for visual quality control. The innovative solution was using generative AI to simulate realistic production images, thus overcoming data scarcity and privacy issues. The key insight is that generative AI can “bootstrap” other AI systems in manufacturing – Bosch expects this to reduce AI solution rollout times from 6–12 months down to a few weeks. In essence, generative AI is not just directly doing tasks, but is creating the training material for other AI, enabling much faster and scalable implementation of automation in plants.
Results: Early results from the pilot plants are very promising. At Bosch’s Hildesheim plant, synthetically generated images were successfully used to train a vision system for electric motor production, and the human eye could not distinguish the AI-generated images from real ones, indicating high realism. By improving its AI tools, Bosch has already seen 15% faster production ramp-up on new lines (through AI optimization of cycle times) and a reduction of certain test process durations from 3.5 minutes to 3 minutes. Executives project that, once generative AI is rolled out globally, the impact will be on the order of “six- to seven-figure cost savings per year, per plant” due to productivity gains. Moreover, roughly half of Bosch’s 240+ factories are already using AI; generative AI will boost these existing solutions to a new level of accuracy and speed, as evidenced by the pilot outcomes.
Timeline: Announced in December 2023, Bosch’s generative AI pilots began in two plants that year. The Hildesheim plant had already incorporated synthetic images into standard inspection systems in 2023. Following successful pilots, Bosch plans a broader rollout in 2024, offering the synthetic data generation service to all its manufacturing locations. This phased timeline shows an initial prove-out in late 2023, with scaling across Bosch’s global manufacturing network in 2024–2025 as a key part of its Industry 4.0 strategy.
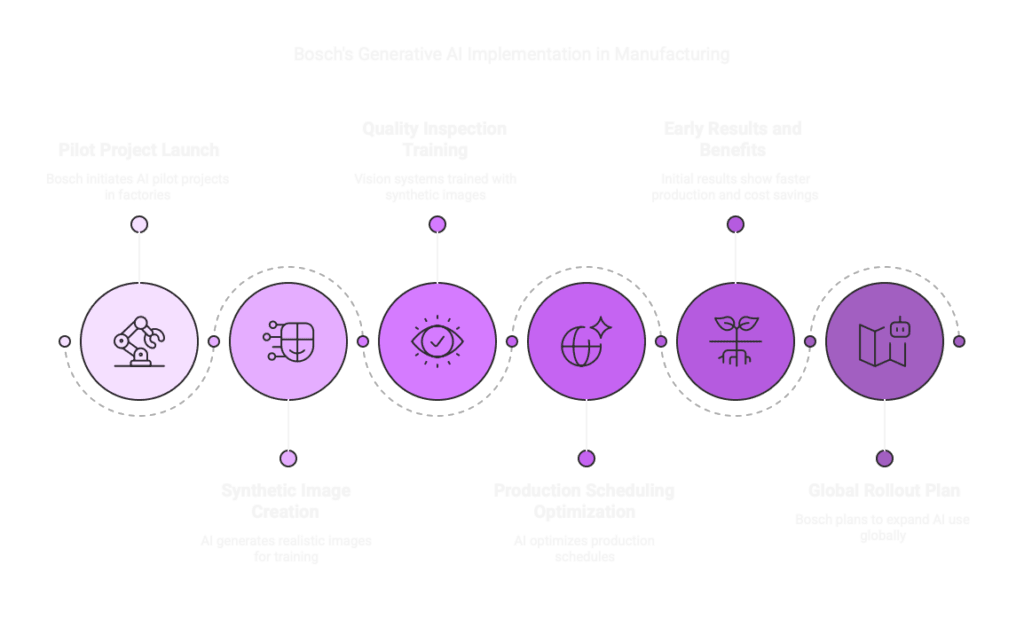
8. Siemens (Industrial Equipment & Automation)
Implementation Summary: Siemens, a global industrial tech company, integrated generative AI “Industrial Copilot” assistants into its automation and engineering platforms. Unveiled in late 2023, the Siemens Industrial Copilot uses a domain-tuned large language model to let factory personnel query and control machines via natural language. For example, an automation engineer can ask the Copilot to generate PLC code for a specific function, or a technician can describe a fault and get troubleshooting guidance. Siemens demonstrated a real customer use-case at the SPS 2023 trade fair: the Copilot was able to produce correct PLC logic from a text description and assist operators in diagnosing machine issues. The system was built in collaboration with Microsoft (leveraging Azure OpenAI) and is embedded in Siemens’ automation software tools, ensuring it has context of the industrial environment.
Key Insight: AI as a skilled assistant on the factory floor: Siemens’ implementation shows that generative AI can be tailored to industrial contexts to serve frontline workers, not just engineers. The key innovation is making AI accessible through a ChatGPT-like interface but with domain expertise – essentially a “ChatGPT for factory workers”. This bridges the gap between complex automation systems and the humans who operate them, by translating plain requests into code or actionable recommendations. A standout insight is how Siemens sees this addressing workforce challenges: the Copilot can reduce the time workers spend on writing/understanding automation code and help less experienced technicians perform like veterans. In short, success comes from integrating AI into existing industrial workflows (e.g., within the HMI or engineering software) so it augments human capabilities in real time.
Results: In prototype deployments, Siemens’ Industrial Copilot has enabled notable productivity gains. Engineers reported that generating PLC function blocks via the AI was **”more quickly” done than manual coding, shortening development cycles significantly. Additionally, machine operators using the Copilot for fault diagnosis have resolved issues faster, as the AI can instantly cross-reference machine data and manuals to suggest likely fixes. While quantitative results are still being gathered (full rollout expected in 2024), Siemens expects the Copilot to cut programming and commissioning time by up to 50% for certain automation tasks, based on internal testing. The AI has also successfully handled natural-language queries in multiple languages, reflecting Siemens’ global workforce needs. Importantly, early users highlight reduced need for specialized programming training, as the AI lowers the learning curve for complex systems.
Timeline: Siemens began developing its industrial LLM assistant in early 2023 amid the surge of generative AI. A working demo was showcased in November 2023 (SPS trade show) with a “tangible customer use case”. In 2024, Siemens is moving from pilot to product, integrating the Copilot into offerings like Siemens Xcelerator and Totally Integrated Automation. A broader commercial launch is anticipated in 2024, making generative AI a standard feature in Siemens’ automation portfolio going forward.
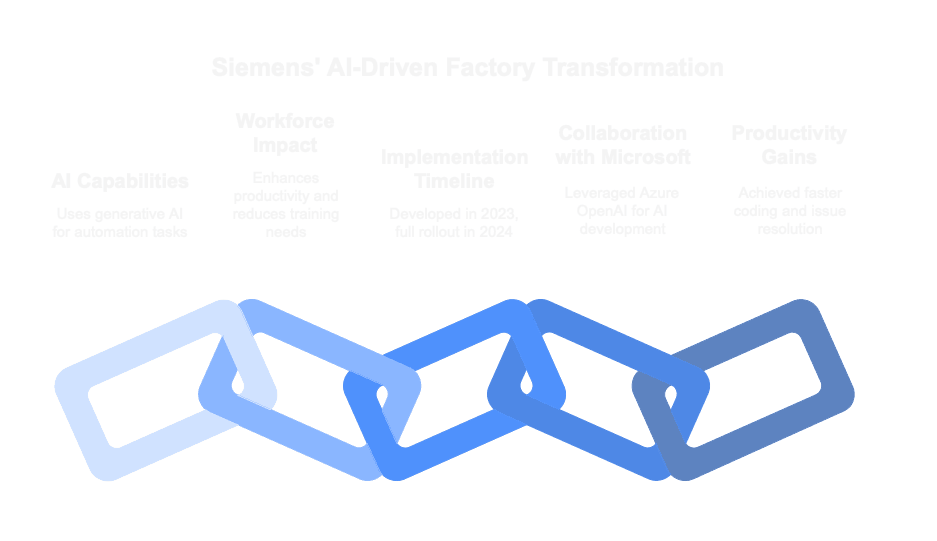
9. Airbus (Aerospace Manufacturing)
Implementation Summary: Airbus applied generative design to re-engineer a cabin partition wall for the A320 aircraft. Starting in 2015, Airbus and Autodesk conducted a feasibility study to use AI-driven generative algorithms (with bionic design inspiration) to optimize the partition – a large wall that separates the passenger area from the galley. Engineers specified requirements such as attachment points, thickness, strength, and of course minimal weight. The generative software then iteratively generated and evaluated thousands of design permutations. Airbus selected a highly organic, lattice-like design and validated that it met all strength and safety criteria. The final design was manufactured by 3D printing a mold and using it to cast the wall in an advanced aluminum alloy.
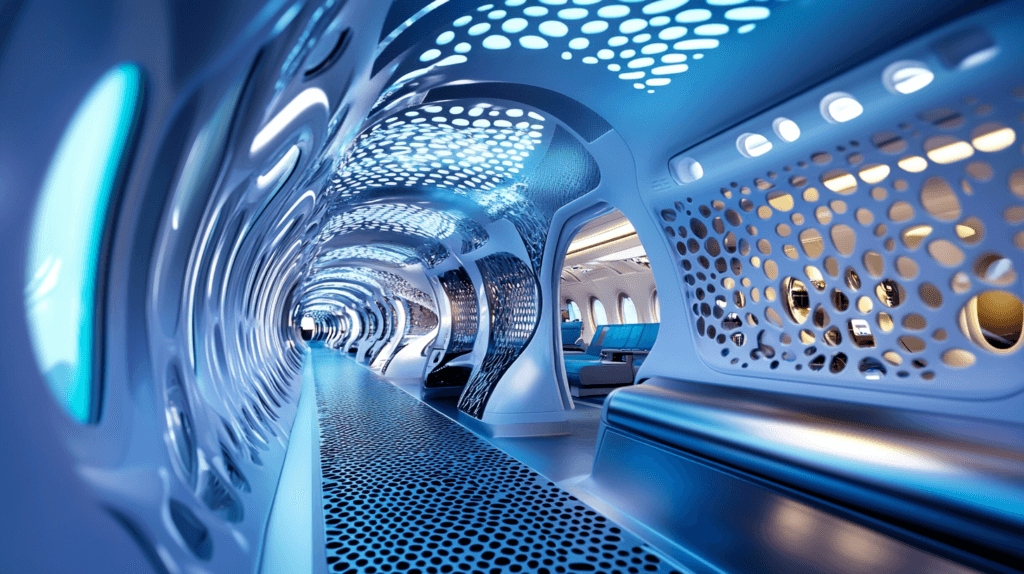
Key Insight: Generative design for extreme light-weighting in aviation: Airbus’s case is a landmark in showing how AI can achieve radical weight savings (nearly 50%) on a certified aircraft component. The key insight is that generative AI, inspired by nature’s efficient structures, can uncover designs far lighter than legacy designs while still complying with stringent aerospace standards. For Airbus, this was also about component consolidation and space savings – the AI-created partition design integrates necessary features in a single structure and frees up space due to its thin, lattice form. It demonstrated that even large load-bearing airplane parts can be re-imagined with AI to improve fuel efficiency (every kg saved matters for airlines). Moreover, this project illuminated the importance of new manufacturing methods (3D printing) in bringing AI designs to reality, foreshadowing a new design-to-fabrication process in aerospace.
Results: The generative-designed A320 partition wall is 45% lighter than the previous design yet just as strong and meeting all regulatory requirements. This weight reduction (almost halving the mass) is an enormous improvement for a single component, contributing to fuel burn savings over the aircraft’s life. The project moved from concept to production: by late 2019, Airbus announced the partition was entering production, becoming one of the first AI-designed components to fly on a passenger aircraft. Besides weight, the design’s innovative geometry is also stiffer in certain directions, offering potential safety benefits in crash scenarios. Airbus engineers noted that such a design would have been impossible to devise without generative AI and modern computing power, illustrating a qualitative leap in design capability. Following the partition, Airbus stated it plans to leverage generative design for other aircraft parts (and in a new engine plant), expecting similar efficiency gains across various structures.
Timeline: The project began in 2015 as a research collaboration. A prototype “bionic partition” was unveiled in 2016, and refinements continued through 2017–2018. By 2019, Airbus had proven the concept and prepared for production tooling. The AI-optimized partition was slated for installation on A320 family jets in the early 2020s after certification. This multi-year timeline underscores the rigorous testing and validation required in aerospace, even as generative AI greatly accelerated the initial design phase.
[9] https://www.hannovermesse.de/en/news/news-articles/airbus-builds-ai-optimized-partition-wall
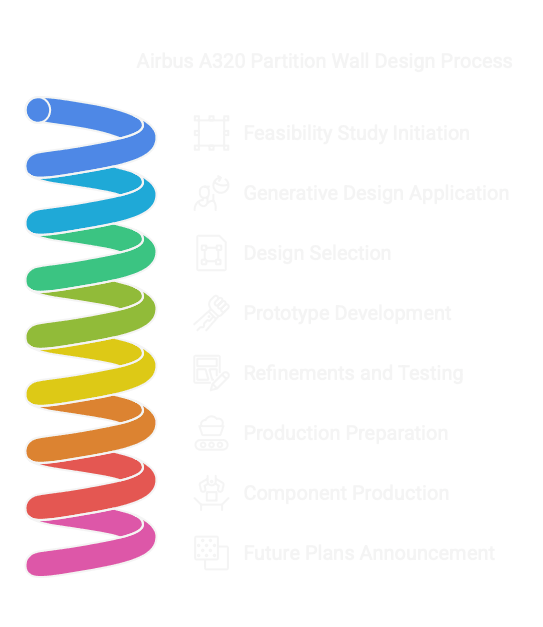
Asia
10. Toyota (Automotive Manufacturing)
Implementation Summary: Toyota, through its Toyota Research Institute (TRI), developed a generative AI system to assist vehicle designers by blending stylistic creativity with engineering feasibility. Announced in June 2023, TRI’s technique allows designers to input an initial sketch of a car design and specific engineering constraints (e.g. drag coefficient targets, chassis dimensions) into a generative model. The AI then produces a multitude of car body designs that not only match the desired style (via text/image prompts like “sleek SUV-like shape”) but also implicitly meet the engineering requirements like aerodynamics and safety regs. This is achieved by combining text-to-image diffusion models with optimization algorithms so that each AI-generated concept is evaluated against physics parameters (like wind resistance) in the loop. In essence, Toyota’s implementation injects engineering “common sense” into generative design for automobiles, to rapidly iterate concepts that are both beautiful and buildable.

Key Insight: Merging creative and engineering design with AI: Toyota’s case offers a blueprint for how generative AI can bridge the traditional gap between automotive stylists and engineers. The standout innovation is feeding engineering constraints (aerodynamic drag, ergonomic dimensions, etc.) into the generative AI process so that the AI’s output is not just a pretty picture, but a starting point grounded in real-world metrics. This greatly cuts down the back-and-forth iterations between design and engineering departments. The key insight is that generative AI can serve as an “amplifier” for designers – giving them viable options that already consider wind tunnel results or chassis fit, thereby reducing the number of design iterations needed to reconcile form and function. It shows the power of multidisciplinary AI: combining visual generation with engineering simulation yields a smarter design tool for complex products like cars.
Results: Toyota’s TRI reported that using this generative technique, designers were able to cut down iterations and time required to converge on a feasible vehicle design. While quantitative metrics were not explicitly disclosed, an example was given where the AI optimized a vehicle’s styling while keeping its drag coefficient within target – something that would normally take many rounds of CFD (computational fluid dynamics) analysis and redesign. By automating that, the design cycle accelerates. Insiders suggest this approach can shorten early-stage concept development by up to 50%, since the AI quickly filters out designs that would be problematic, allowing designers to focus only on viable concepts. Moreover, the quality of design options improved: the AI yielded novel shapes that met performance thresholds, expanding the creative palette. Toyota also leveraged generative AI in a marketing context (“Lexus art generator” at an auto show) and is eyeing in-vehicle AI assistants, indicating broad benefits beyond engineering.
Timeline: The generative design assistance was developed in late 2022 and publicly unveiled in June 2023. It was demonstrated with concept examples at that time. Throughout 2023, Toyota has been integrating this AI technique into its vehicle design workflow, initially as a supportive tool for designers to experiment with. Going forward, Toyota plans to incorporate generative AI into production vehicle programs, using 2023’s prototype as the foundation. The TRI papers published in 2023 suggest that future Toyota models, especially EVs with new design freedoms, could see styling influenced by AI-generated concepts within the next design cycles (2025 and beyond).
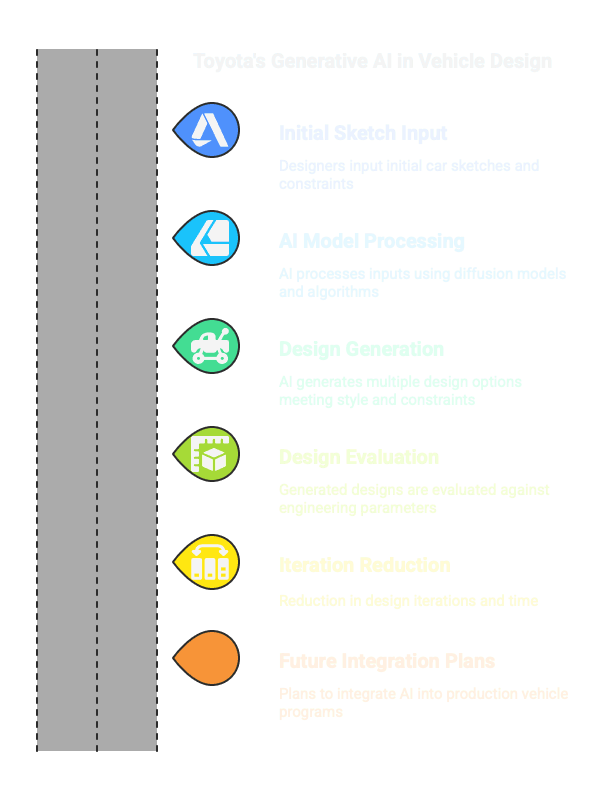
Synthesis
Common Implementation Patterns
Across these 10 case studies, several clear patterns emerge in how generative AI is being applied in industrial manufacturing:
- Generative Design for Parts and Products: Many organizations (GM, Stanley Black & Decker, GE, Airbus, Toyota) used generative AI to create optimized designs for physical components. These projects commonly target weight reduction and performance improvement, with AI algorithms exploring complex shapes under defined constraints. The outcomes were strikingly consistent – AI found designs that cut weight by 30–60% while meeting or exceeding strength requirements. This pattern shows up in automotive, aerospace, and tool manufacturing alike, indicating a broad applicability of AI-driven lightweighting and part consolidation. Generative design is often paired with additive manufacturing (3D printing) as the enabler to fabricate AI’s free-form geometries.
- AI Co-pilots for Engineers and Operators: Several companies (Rockwell, Siemens, Lockheed) implemented generative AI as a supportive assistant in technical workflows. This pattern entails embedding large-language models or similar AI into software that engineers or factory workers use, effectively creating a “co-pilot” for tasks like programming controllers, diagnosing machinery, or analyzing data. For example, both Siemens and Rockwell launched natural language code generation assistants to help build automation logic faster. Lockheed built an internal ChatGPT-like tool to aid its staff in coding and data tasks securely. In all cases, the AI isn’t acting autonomously but rather augmenting human work – providing suggestions, answering questions, and handling grunt work (coding, searching) so humans can focus on higher-level decisions.
- Synthetic Data and Training Acceleration: A newer pattern shown by Bosch (and hinted by others) is using generative AI to create synthetic training data or simulations to speed up AI deployment. In Bosch’s case, generative models produce realistic images of parts to train machine vision systems, eliminating the wait to gather real defect images. This approach – using one AI to help train another – suggests a pattern where generative AI is leveraged to overcome data scarcity in manufacturing (for quality inspection, predictive maintenance, etc.). It dramatically accelerates the rollout of AI solutions by providing abundant, varied training examples in a short time. This synthetic data generation pattern may expand to simulating sensor readings, factory scenarios, or even customer demand patterns using generative models.
- Pilot Projects Leading to Scale-Up: Virtually all these case studies started as targeted pilot implementationsin a specific domain (a single part, one production line, one internal department) to prove value before wider adoption. For instance, Stanley’s team applied generative design to just one tool component as an experiment; Bosch trialed generative vision at two plants before planning a global rollout; Rockwell and Siemens prototyped their AI copilots with select users in 2023. A pattern is that companies first tackle a well-defined problem where generative AI could make a big impact (lighten this bracket, assist this coding task) and use that success to justify broader implementation. After the pilot’s concrete results, we see organizations move to integrate generative AI into standard processes or product offerings (e.g., Airbus moving the AI-designed partition into production, or Toyota embedding AI in its design workflow). This phased approach – pilot, prove, then scale – is a common implementation trajectory.
Success Factors
Analyzing the successes, several factors stand out as critical in achieving positive results from generative AI in manufacturing:
- Clear Objectives & Metrics: Each successful case had a well-defined goal and yardstick for success – lighter weight by X%, reduce design time by Y%, improve yield by Z%, etc. This focus guided the AI implementation. GM and Airbus, for example, set explicit weight reduction targets (and AI exceeded them). Bosch aimed to shrink AI deployment time from months to weeks. Because the objectives were clear, teams could measure and celebrate concrete results (e.g. “60% lighter tool achieved” or “15% faster ramp-up”) which helped in securing buy-in for expansion.
- Domain Constraint Integration: A crucial success factor was integrating domain knowledge and constraints into the generative AI process. The Toyota case highlights this – by baking engineering constraints (aerodynamics, safety parameters) into the AI model, the outputs were immediately viable for production. Similarly, Airbus provided the generative software with precise specifications (thickness, attachment points, etc.), and Siemens tuned its LLM with factory automation context. This melding of AI with engineering rules prevented “wild” outputs and ensured the AI’s creativity was channeled towards practical, acceptable solutions. Success was much more likely when the AI’s sandbox was well-defined by real-world requirements.
- Human-in-the-Loop and Multi-disciplinary Teams: In all cases, human expertise remained vital. Teams that blended AI specialists with veteran engineers/designers had the most success. For instance, Stanley’s engineers guided the AI by inputting the right criteria and then evaluated the AI options. At GE, experts in aerodynamics worked alongside Autodesk’s AI team to refine designs. Rockwell’s approach explicitly keeps the engineer in charge, calling the AI a “co-pilot, not an autopilot”. This human-in-the-loop model ensured that AI suggestions were vetted and improved by domain experts, combining the best of both. Companies that treated generative AI as a collaborative tool rather than a black box had smoother adoption and better outcomes.
- Top Management Support and Vision: Many of these initiatives enjoyed strong backing from leadership who championed AI innovation. Bosch’s board-level support is evident (with quotes from its chairman about AI’s central role). Siemens’ and Rockwell’s presidents publicly drove the AI co-pilot vision. Lockheed’s AI Factory had executive endorsement to train thousands of staff. This support provided the necessary resources (funding for pilots, investment in infrastructure like supercomputers or 3D printers, training programs) and also signaled to the organization that AI is a strategic priority, not just a lab experiment. Such vision helped overcome internal inertia and siloed thinking.
- Partnerships and Ecosystem Leverage: A subtle but important factor was that companies partnered where needed instead of going it alone. GM with Autodesk, Stanley with Autodesk, Siemens and Rockwell with Microsoft, Airbus with Autodesk, etc. By leveraging cutting-edge AI technology from specialized providers (or academic collaborations), industrial firms accelerated their implementations. They combined their deep manufacturing knowledge with partner’s AI expertise. This reduced development time and risk, and ensured access to the latest algorithms (e.g., using Azure OpenAI’s GPT-4 for industrial coding, or Autodesk’s generative engine for design). These partnerships, often between traditional manufacturers and tech firms, were a key success factor in getting generative AI up and running effectively.
Challenges & Solutions
Implementing generative AI in manufacturing did not come without challenges. The case studies reveal several common hurdles and how organizations addressed them:
- Ensuring Feasibility and Safety: One big challenge is that generative AI might propose designs or solutions that are optimal in theory but not practical or safe to implement. Aerospace and automotive firms faced this head-on – a wild AI-designed part might be un manufacturable or fail certification. The solution was to tightly integrate engineering checks and balances. Toyota’s approach of building constraints into the AI is one solution. Airbus and GM conducted extensive simulations and physical testing of AI-generated designs to verify performance and safety before approval. In Siemens’ case, the AI co-pilot’s code suggestions are tested in simulation sandboxes before being used on real machines. Essentially, companies set up a robust validation pipeline for generative outputs – using CAE (computer-aided engineering) tools, test labs, or expert review – to filter out any AI mistakes or risky suggestions. This mitigated the feasibility challenge.
- Data and Training Limitations: For AI models to be useful, they need data (for training or context). In manufacturing, quality data can be limited or proprietary. Bosch specifically tackled the lack of diverse training images for defect detection; the solution was generative synthetic data to supplement real datasets. More generally, companies overcame data scarcity by using pre-trained foundation models (as Lockheed did with a base model on a supercomputer, then fine-tuned internally) or by starting with small pilots to gather data and build momentum. Another data challenge is sensitivity: manufacturing often involves trade secrets or regulated data (e.g., Lockheed’s classified projects). The solution here was deploying generative AI in secure on-premises environments or private clouds (Lockheed’s internal LLM, Bosch’s in-house AI platform) so that no sensitive data was exposed to public AI services. This allowed use of AI where data privacy was non-negotiable.
- Workforce Adoption and Skill Gap: Introducing AI co-creators can be disruptive to engineers and operators – some might resist or not trust the AI, and others might lack skills to use it effectively. Several solutions emerged: user-friendly interfaces and training were key. Siemens and Rockwell deliberately made their AI interface natural language-based, lowering the barrier for factory personnel to try it. Companies also invested in upskilling programs – e.g., Lockheed trained 8,000 staff on its AI platform. Early success stories from pilots also helped win over skeptics; when engineers see that AI can save them time or produce a design that meets specs, their trust increases. Organizations often started with volunteers or digitally savvy teams to build internal champions, who then advocated the AI’s benefits to peers.
- Integration with Existing Processes: Another challenge was incorporating generative AI into legacy workflows and software. Manufacturing processes are mature and often standardized (CAD systems, PLM workflows, etc.), so an AI solution can’t exist in a vacuum. The solution was integration and custom development: for example, GM plugged Autodesk’s generative design into its existing CAD environment so designers could seamlessly use it. Bosch integrated synthetic image generation into its vision system pipeline, not as a separate tool. Siemens built its Copilot into the automation software that engineers were already using. This tight integration meant workers didn’t have to leave their familiar environment to use the AI – it became just another feature in their toolset. Also, IT departments ensured that AI outputs could be captured in regular data management systems (for traceability, revision control). Such integration work was critical to move from one-off pilot to routine usage.
- Managing AI Limitations (Hallucinations, Errors): Generative AI, especially language models, can sometimes produce incorrect or nonsensical outputs (“hallucinations”). In an industrial context, an error could be costly or dangerous. Companies addressed this by keeping a human in the loop for verification (as noted) and by domain-specific fine-tuning. Rockwell, for instance, constrained their Co-pilot with Rockwell’s own verified code library to prevent it from suggesting non-compliant logic. Many implemented a requirement that AI suggestions be reviewed just like a junior engineer’s work. Over time, as the AI proved reliable on routine tasks, it earned more trust for autonomous suggestions, but critical decisions always had human oversight. This pragmatic approach balanced embracing AI’s efficiency with caution appropriate to high-stakes manufacturing.
Impact Assessment
The introduction of generative AI in these manufacturing organizations has led to significant impacts, both quantitative and qualitative:
- Efficiency Gains and Time Savings: Perhaps the most immediately felt impact is in time reduction for various processes. Design cycles that normally took months were cut down to weeks or days in pilots. Boeing saw major reductions in component design and testing time. Lockheed’s 8,000 engineers using generative code assistants are speeding through software and analysis tasks much faster than before (some reporting tasks done in hours vs days). Siemens expects programming time for factory automation to drop substantially (their quote: “reduce time… particularly important given the shortage of skilled workers”). In Bosch’s case, deploying AI solutions in production is now projected to be 4–5× faster using generative approaches. These efficiency gains translate to faster time-to-market for new products, faster factory commissioning, and more agile responses to design changes or production issues.
- Performance and Product Improvements: Generative AI has directly improved the performance of products and processes. In product design, we see lighter, stronger components – e.g., 40–60% weight reduction in parts (GM’s bracket, Stanley’s tool, Airbus’s partition). For aerospace and auto companies, this yields more fuel-efficient, higher-performing end products (cars with better fuel economy or EV range, planes with lower fuel burn). GE’s engine frame got both lighter and achieved a 91% reduction in pressure drop, potentially improving engine efficiency and longevity. On the process side, AI-optimized workflows led to quality and output gains: Bosch reported a 15% reduction in cycle time during line ramp-up and eliminated certain inspection bottlenecks. Unilever (via a Rockwell mention) saw 50% reduction in product “giveaway” waste by using AI in filling lines. So the impact is not just doing things faster, but doing them better – products are higher quality or more optimized, and processes are more efficient, yielding less waste and scrap.
- Cost Savings: The combination of efficiency and performance gains brings substantial cost benefits. Bosch anticipates each AI-enhanced factory will save on the order of hundreds of thousands of dollars annually. Part consolidation and weight reduction save material and production costs – GE’s new engine frame design reduced material waste by 95% in that part’s fabrication, and consolidating 150 parts into 1 lowers assembly labor and inventory costs. Several companies also noted that predictive maintenance enabled by AI (though not always generative) avoids expensive downtime. While not every case provided a dollar figure, an illustrative data point: Siemens in other studies found AI in design can cut development costs 10–15%, and AI in operations can reduce unplanned downtime by 30–50%, which for a large plant is millions saved. Furthermore, accelerating R&D cycles (as Toyota experienced) means cost savings in engineering man-hours and an opportunity cost gain by reaching market sooner.
- Workforce Impact – Productivity and Upskilling: Rather than displacing workers, these generative AI implementations mostly augmented the workforce and made their jobs easier or more interesting. Engineers and designers could offload tedious tasks (like exploring dozens of design iterations or writing boilerplate code) to the AI, and focus on higher-level creative or analytical work. This boosted productivity per employee. Lockheed’s thousands of engineers can tackle more projects now with the AI assist. At the same time, companies invested in upskilling workers to use AI tools – effectively raising the technical competency of their workforce. There is anecdotal evidence of improved employee satisfaction when AI handles drudge work and when workers see faster success in their projects. That said, these changes also require change management; some cases undoubtedly faced initial fear of job impact, but the narrative in these companies has been that AI is a tool foremployees. For instance, Rockwell’s and Siemens’ messaging frames AI as helping engineers do their job better, not replacing them, and that seems borne out by the adoption rates.
- Innovation Capacity: An often overlooked but critical impact is the boost to innovation capacity. By dramatically reducing the cost (in time and effort) of exploring new ideas, generative AI lets companies iterate more and take more “shots on goal.” GM can explore hundreds of part designs in the time they used to manually model a handful. Nike’s designers, in another example, generated “dozens of concepts” for a new shoe in hours using AI image generators, fueling more creativity. This means R&D teams can be bolder and more experimental, knowing AI helps fail fast and cheap. The result is a potential pipeline of more innovative products and solutions. Several executives alluded to this: Toyota’s team explicitly said it’s using AI to “amplify designers” and allow more creative exploration without sacrificing engineering rigor. Lockheed’s AI Factory is intended to push the frontiers of what their engineers can do, suggesting a long-term innovation play. In summary, generative AI is expanding the solution-space in engineering and unlocking fresh possibilities, which could lead to breakthrough products (e.g., Airbus’s radical new partition design) that give companies a competitive edge.
Future Directions Based on These 10 Case Studies
Drawing on these case studies, we can project several future directions for generative AI in industrial manufacturing:
- Broader Adoption and Standardization: What was innovative in 2023–2024 will become standard practice by 2025 and beyond. We can expect generative design to move from a few hero projects to a normal step in the design of many components. Companies like GM and Airbus, having proven it on one part, will apply it to dozens of parts across new models. Similarly, AI copilots on the shop floor (like Siemens’ and Rockwell’s) will likely be rolled out across many factories and even become a selling point for industrial software platforms. According to a Capgemini survey, 41% of A&D organizations are already piloting generative AI for design – this figure will grow, and pilots will turn into production systems. We may see industry standards emerge for validating AI-designed parts or for certifying AI-generated code in safety-critical systems, as regulatory bodies catch up with these technologies.
- Integration with the Digital Twin & IoT Ecosystem: Future implementations will link generative AI with digital twins (virtual replicas of factory equipment or products) and IoT data streams. This means AI could dynamically generate solutions in real-time as conditions change. For example, a generative AI agent might tweak a production line layout or robot path on the fly in response to bottlenecks (a kind of generative optimization of operations). Some groundwork is already there – NVIDIA and others are talking about “AI Factories” and digital twin simulations (as with Foxconn’s plans). We anticipate generative AI will be used to run thousands of “what-if” simulations in a digital twin of a factory, then propose the optimal configuration or schedule, effectively performing autonomous continuous improvement. This would bring generative design principles to process engineering and operations, not just product design.
- Enhanced Collaboration Between AI and Humans: In the future, the boundary between human and AI contributions in design and problem-solving will blur further. We’ll likely see more intuitive interfaces – voice-controlled AI assistants on factory floors, AR glasses where a worker sees AI-generated suggestions in context (e.g., highlighting which part to pick or how to assemble based on AI guidance). The co-pilot concept will mature: an engineer might start designing a product simply by conversing with an AI that draws CAD models in real time. Companies will refine how they leverage human creativity alongside AI’s brute-force exploration. The “Generative AI + human expert” teams will become the norm in engineering departments. We may even see multi-AI collaboration: one AI generates designs, another critiques for manufacturability, and the human makes the final call – a true hybrid design team.
- Addressing New Challenges: Data Governance and Ethics: As generative AI becomes more ingrained, companies will need to formalize policies around its use. Intellectual property questions will arise (e.g., who owns an AI-generated design? Is it patentable?). Companies might develop curated proprietary training datasets to maintain a competitive edge – for instance, train their generative models on decades of their own designs and field data, creating an AI deeply specialized in their products (imagine a “Toyota GPT” for car design, beyond the prototype we saw). There will also be a focus on AI ethics and safety – ensuring an AI doesn’t introduce a subtle flaw that goes unnoticed. Hence, future directions include more sophisticated validation tools (perhaps AI systems that verify other AIs) and traceability for AI decisions (audit logs of why the AI chose a design). We might see regulatory oversight increasing for AI-designed critical components, meaning companies will invest in explainable generative AI to satisfy regulators.
- Extended Use Cases – From Supply Chain to Customer Interface: The case studies mostly center on design and production, but generative AI’s reach will extend end-to-end. In supply chain management, generative AI could be used to simulate and predict demand or to design optimized logistics networks (generating scenarios for sourcing, inventory placement etc., then proposing the best one). Some manufacturers are already exploring using GPT-like models for generating shop floor instructions, maintenance manuals, or even training materials in multiple languages on the fly. On the customer side, generative AI can enable mass customization – customers might co-create product variants by describing their preferences to an AI, and the system generates a manufacturable design automatically. We see early hints of this in automotive (customizing trim via AI) and consumer goods. Another future use case is in sustainability: generative AI could be tasked with designing products or factory processes that minimize carbon footprint or material waste, balancing environmental constraints creatively. This could accelerate the development of greener technologies (new battery designs, lighter packaging, recycled material usage optimized by AI). In sum, generative AI will become a pervasive assistant not just in the core factory, but across the manufacturing enterprise from R&D to customer engagement.
In conclusion, these 10 case studies collectively show that generative AI is no longer experimental in manufacturing – it’s delivering tangible benefits in cost, time, quality, and innovation. Companies that have embraced it are already outpacing others in product development and operational efficiency. Moving forward, generative AI is poised to become a backbone technology in Industry 4.0, enabling factories and design centers to be more agile, inventive, and efficient than ever before. The trailblazing implementations from 2023–2025 will guide broader adoption, as best practices crystallize around human-AI collaboration, data management, and scaling strategies. Manufacturers that learn from these pioneers – focusing on clear objectives, integrating domain knowledge, and keeping humans in control of the narrative – are likely to reap significant competitive advantages in the years ahead.
Discover Generative AI in AI Assistants on ChatGPT
Disclaimer: This content was generated using generative AI technologies as part of exploratory research on industrial AI use cases. While we have strived for maximum accuracy, some information, references, or details may contain inaccuracies or “hallucinations” inherent to current AI models. These articles are provided for informational and educational purposes only – we recommend verifying critical information with original sources before making any decisions based on this data.
© 2025 OneDayOneGPT – Content produced via OpenAI Deep Research